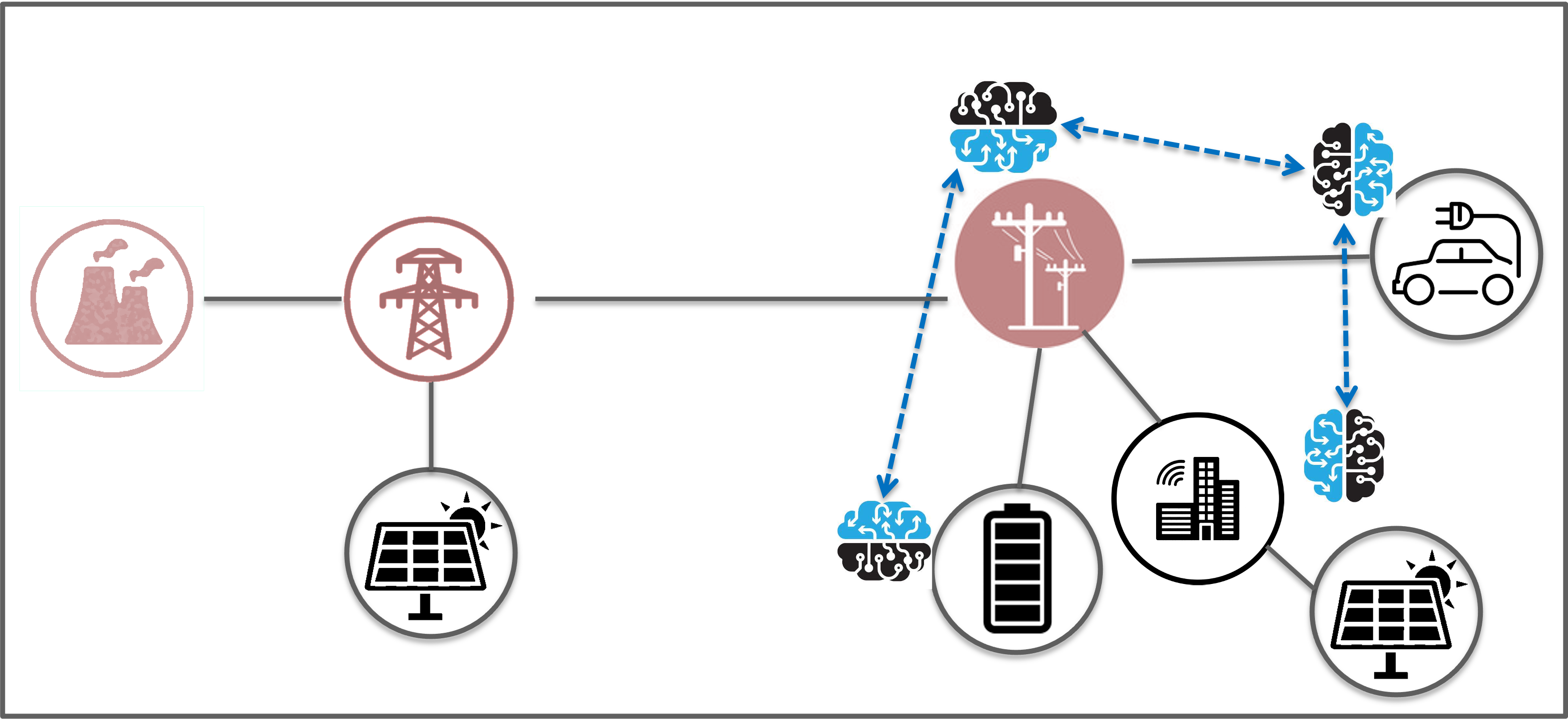
ML@GridEdge: Distributed Machine Learning Solutions for Coordinating Distributed Energy Resources at the Edge of the Power Grid
Smart buildings and connected communities are the cornerstones of future sustainable power grids. Tomorrow’s energy communities and the resilient operation of the energy infrastructure hinges on accurate prediction of buildings’ temporal energy presumption (production and consumption). Traditional prediction models primarily leverage historical information (such as energy demand) at the grid or building level. This is mainly due to data sharing hesitance which originates from privacy concerns of building managers and occupants. This project intends to develop a novel distributed machine learning model for predicting the temporal energy needs of future connected communities. The proposed multi-agent approach leverages centralized oversight of a central aggregator to inform distributed collaboration among each building, taking advantage of additional private information that is not shared with the aggregator. The proposed solution lends itself well to a multi-agent structure of connected energy communities where agents are willing to collaborate to improve their prediction accuracy. [Press Release].